In the world of big data and analytics, businesses rely heavily on efficient data storage and management systems to make informed decisions. Two major solutions dominate this landscape – data lakes and data warehouses. Choosing the right solution between the two is critical, as it affects not only raw data and storage but also the ability to perform data analysis and unlock business intelligence(BI). We will examine the distinctions, advantages, and applications of both data lakes and data warehouses to assist you in selecting the best solution for your business requirements.
Key Takeaways
- Data warehouses handle structured and historical data, while data lakes store raw, unstructured, and semi-structured data for greater flexibility.
- Data warehouses focus on preprocessed data for analysis, while data lakes allow for on-demand processing, making them ideal for machine learning and big data projects.
Understanding Data Warehouses
Data warehouses are centralized repositories designed to store, manage, and analyze large volumes of structured and partially structured data from various data sources. They play a crucial role in analytical processing, enabling organizations to extract insights from incoming data and generate reports. Key characteristics of data warehouses include:- Structured Data: Data is organized within a set schema, which simplifies the process of querying and analysis.
- Historical Data: Data warehouses typically store past data, allowing for trend analysis and forecasting.
- Data Accuracy: Data is cleansed and standardized to ensure accuracy and consistency.
- Data Management: Data is ETL (Extract, Transform, Load) processed before being stored, making it ready for analysis.
Understanding Data Lakes
Data lakes are large, scalable repositories that store vast amounts of raw data in their native format until it is needed for analysis. It is a relational database that is built to support a diverse range of data types, encompassing structured, partially-structured, and unstructured data. Key characteristics of data lakes include:- Raw Data: Data stored in its original format, preserving its integrity and flexibility.
- Unstructured Data: Data lakes can handle unorganized data, such as text, images, and audio files.
- Semi-Structured Data: Data lakes can also be stored in JSON and XML.
- Data Storage: Data lakes often leverage cloud-based storage facilities for scalability and cost-effectiveness.
- Data Science: Data lakes are well-suited for data mining projects, including ML and predictive analytics.
Key Factors in Choosing Between a Data Lake and Data Warehouse
The decision to use a data lake is influenced by several factors, including the organization’s specific needs, data characteristics, and technical capabilities for storing the data elsewhere. Here are some key considerations:- Data Type: If your data is primarily structured and historical, a data warehouse might be a good fit. However, if you deal with a variety of data types, including unstructured and semi-organized data, a data lake solution offers more flexibility.
- Data Processing: A data warehouse might be more efficient if you require extensive data processing and transformation before analysis. However, a data lake offers more flexibility if you prefer to process data on demand.
- Scalability: Both data lakes and data warehouses can be scaled to accommodate growing data volumes. However, data lakes often leverage cloud-based storage solutions, making them more scalable and cost-effective in the long run.

Data Lakehouse vs. Data Warehouse
A data Lakehouse combines the strengths of data lakes and data warehouses, offering a unified platform for both structured and unstructured data. Unlike traditional data warehouses that are optimized for structured data and analytics, Lakehouses provide flexibility for data scientists and engineers to work with varied data types using both SQL and data science tools. Benefits:- Unified Data Repository: Store both organized and unorganized data in the same system, simplifying your data handling.
- Improved Data Quality and Integrity: Enforce data quality controls while maintaining the flexibility to store irregular data.
- Cost Efficiency: Similar to data lakes, data Lakehouses allow for scalable, cost-effective storage while also supporting high-performance analytics like data warehouses.
- Faster Processing: By combining the best features of both solutions, a data Lakehouse ensures faster processing and analysis of both historical and real-time data.
Why Work With Data Lakes?
Data Lakes provide numerous benefits compared to conventional data warehouses, making them a compelling choice for data engineers in various organizations. To enhance data accessibility and streamline their data management strategy, organizations often implement data warehouse solutions and data lake solutions as centralized repositories that enable them to collect data from various data sources, efficiently store semi-organized data, and generate insightful reports based on their relational data. A few reasons why it might be preferable to work with data lakes:- Flexibility: Data lakes can accommodate a wide variety of data types, including unstructured and semi-organized data. This flexibility enables organizations to collect and store data from multiple sources without the constraints of predefined schemas.
- Scalability: Data lakes are highly scalable, making them suitable for organizations with large and growing data sets. Cloud-based storage systems can be easily scaled up or down to meet changing storage needs.
- Cost-Effectiveness: Data lakes are often more affordable than traditional data warehouses, particularly for businesses managing large data sets. Cloud-based storage solutions use pay-as-you-go pricing, lowering initial expenses and removing the requirement for long-term capacity planning.
- Innovation: Data lakes are well-suited for machine learning projects, enabling organizations to explore new opportunities and drive innovation. The flexibility and scalability of data lakes make them ideal for experimenting with different collected data evaluation techniques and models.
Conclusion
If you require real-time analysis and BI reporting on rational data, a data warehouse is a better fit. However, if your data is diverse and includes large volumes of raw form and unorganized data, or you’re exploring machine learning and data mining projects, a Data lake is your go-to choice. By recognizing the distinctions and advantages of both, you can select the most effective data maintenance and management strategy for your organization, ensuring efficient storage and analysis of your accessible data.Frequently Asked Questions (FAQs)
1.Can I store both structured and unstructured data in a Data Warehouse?
No, Data Warehouses are optimized for organized data. For unorganized or semi-organized data, you would need to use a data lake.2. Is a Data lake more suitable for real-time analytics?
While Data lakes can store real-time and past data, they are generally more suited for batch processing. For real-time data analytics, a cloud data warehouse may provide faster querying capabilities.3. Which solution is more cost-effective for large data volumes?
Data lakes are generally more affordable for storing significant volumes of varied data because they do not necessitate costly preprocessing.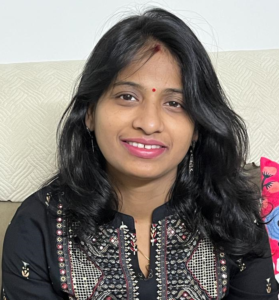
Rasmita Patro
Author