However, a data product is different from data as a product. A data product uses the data to help achieve the product’s objective, whereas data as a product views the data as the real product.
The following article explains the distinction between “data products” and “data-as-a-product” and outlines the key factors required to implement a data product strategy in 2023 successfully.
What Is A Data Product?
A data product is a technology product or element that relies on data as its primary facilitator to achieve its objective. It includes platforms or tools that analyze data and provide results, empowering decision-making with insights from data products.
The economic value of a data product lies in the competitive benefits they offer through upgrading products or services based on trusted data, website engagement, surveys, and other data assets via data-driven research.
A data product consists of its definition, metadata, and the resultant dataset- for example, a customer segmentation tool or a recommendation engine in e-commerce.
Data management service providers can provide more value to companies by delivering customized solutions that optimize data integration, governance, and analysis.
Metadata And Dataset Instances
Metadata is an essential component of data product management as it represents how the data product is handled and provides information to the data teams. It includes-
- Static metadata- It encompasses all necessary databases and tables that collect data from the product.
- Active metadata- It deals with data consumption and performance.
- Synchronization rules- These specify when and how data is synchronized with data repositories.
- Algorithms- They transform, process, enrich, and mask raw data.
- Access controls control credential assessment and authentication, making metadata crucial for supporting data governance initiatives, regulatory compliance standards, and data management procedures.
By leveraging Data Life Cycle Management Services from 5Data Inc., organizations can transform their data products into enterprise-wide value and derive actionable insights to support decision-making.
Data Mesh Architecture
The data mesh paradigm was introduced to respond to organizations’ challenges when scaling their data architecture.
In the context of data products, data mesh refers to the practice of treating data as a first-class citizen and deploying data products that are self-contained, self-describing, and discoverable.

Types of Data Products
A data warehouse is a data engineering product that combines all data types in one place. Therefore, data products can be classified in various ways based on the type of data and the function they provide:
- Raw or Unprocessed Data: This is the most basic type of data collected by a system and has not been processed or used in any way. However, data scientists can still process this sensitive data to increase its value and transform it into a data product.
- Derived Information: Created by processing the raw data and performing additional procedures to make it more understandable for the domain data teams.
Derived information is more personalized and provides more insights than raw data.
- Algorithms: Data products that use algorithms to process and analyze data fall under this category. It includes:
- Decision Support (Insights): These data products use algorithms to process data and provide responses to the data consumers, which can be used to draw inferences and make decisions. However, they still require some level of human interaction to function.
- Automated Decision-Making: These data products use algorithms to provide business outcomes without human interaction or support. They can be viewed as one-size-fits-all algorithms.
By understanding the different types of data products, data engineers can better utilize their derived data products to create the required data models.
Examples Of Data Products
- Microsoft Office 365: A productivity suite that uses data for internal users and document creation to improve its tools and features.
- Netflix: A streaming service that uses real-time data on user viewing habits to personalize content recommendations and improve its content offerings.
- Google Search: Uses complex algorithms to analyze and index web pages, allowing users to find relevant information online quickly.
Understand Data As A Product
Data as a product refers to treating data as a valuable and strategic asset that can be analyzed and packaged for consumption by external and internal users.
Fundamentally, Data as a product is a concept or methodology that describes how data teams may add value to their organizations. The common consensus is that implementing product management practices into a centralized data team will make data work more valuable and scalable, two attributes that have long been absent in the data community.
It is essential to implement best practices by the Data collection and Data Management Service Providers, such as ensuring data quality, proper governance, security, and accessibility and providing a user-friendly interface for internal customers.
Benefits Of Using Data As A Product
- Improved Customer Experiences: Data as a product can help businesses better understand their customers and provide personalized experiences that increase business value, customer satisfaction, and loyalty.
- Improved Data Governance: Data as a product can improve data governance by ensuring data quality and transparency.
- Collaboration: It can facilitate collaboration between the data team’s role and data consumers, enabling them to share data insights and work towards company domain expertise.
- New Revenue Streams: By treating data as a product, business users can monetize their data assets by selling insights to customers or partners, creating new revenue streams, and improving their bottom line.
Implementing Data As A Product Strategy
- Collaborate with stakeholders early on to obtain consensus and ensure data products align with their business units.
- Adopt a product management mentality, using established practices such as scoping and iteration for various business domains.
- Implement self-service technology to make data more accessible and enable the best team structure to create data products.
- Emphasize data quality and dependability while collaborating with the primary data consumers.
- Choose the best team structure of data analysts for your data processing needs to meet business objectives quickly while maintaining control over data integrity and management.
According to a recent market study, the Global Product Analytics Market was valued at USD 10.87 billion in 2022 and is expected to reach USD 49.31 billion by 2030 at a Compound Annual Growth Rate (CAGR) of 20.80%.
Overview
Data products are specific deliverables data scientists create, such as reports, dashboards, or visualizations, that provide valuable insights to stakeholders.
Meanwhile, data as a product is a broader approach that involves treating data as a valuable commodity and applying product management practices to its development, management, and distribution. This approach recognizes data as a strategic asset that help in deploying reusable data asset to drive innovation aligned with your business success.
5Data Inc., the Data Collection and Data Management Service Provider, understands your product management principles and provides the best solution for your Data Lifecycle Management (DLM) challenges. Our data team provides greater insights into your data by providing the best Data Lifecycle Management Services to grow your business and stay ahead of the curve.
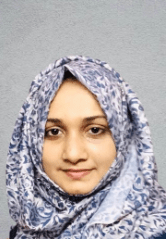