What we cover in this blog
Understanding Hybrid Data Models
A hybrid data architecture integrates the capabilities of data lakes and data warehouses, creating a unified data bank and management solution. This approach allows organizations to store, manage, and process different types of data, including structured and semi-structured data while ensuring efficient data retrieval and analytics.
What is a Hybrid Data Lake?
A hybrid data lake is an advanced data retention system that blends the features of cloud-based and on-premises data storage solutions. It combines the flexibility of data lakes with the structured capabilities of data warehouses, providing a comprehensive environment for handling big data. Hybrid data lakes allow organizations to store raw data in object storage while ensuring structured data is accessible for data intelligence and transactional applications.
What is a data warehouse?
A data warehouse is a data retention system designed for storing and analyzing organized data. It is typically used to support business intelligence (BI) and analytics applications. Data warehouses are designed to deliver quick query performance, though they may incur high maintenance costs.
Need For Both Data Lakes and Data Warehouses
Organizations need both data lakes and data warehouses for different business and analytical needs. A data warehouse is optimized for structured data and predefined schemas, making it ideal for business analysis and historical reporting. Conversely, data lake houses support unstructured and semi-organized data, which is critical for machine learning, data exploration, and data mining.
By integrating both solutions into a hybrid data structures, organizations can:
- Improve data entry and availability
- Enhance data protection and governance
- Reduce expenses by storing historical data in a cost-effective manner
- Support a wide range of analytics and data intelligence functions
Key Components of a Hybrid Data Model
Behavioral analytics focuses on analyzing the actions of users, revealing patterns and trends that can inform business strategy. By combining behavioral data with survey objectives, organizations can:
- Data Storage: The architecture integrates object storage for raw data and structured databases for transactional data.
- Data Ingestion: A robust data pipeline enables seamless ingestion of incoming data from multiple sources.
- Data Integration: Ensures data consistency and availability across cloud environments and on-premises systems.
- Data Analysis: Advanced processing capabilities facilitate data intelligence, machine learning, and data analytics.
- Data Governance and Security: Centralized governance ensures data accuracy, access control, and compliance.
- Data Access and Retrieval: Efficient querying mechanisms provide fast access to structured and unstructured data.
By carefully evaluating these factors, you can select a hybrid data model solution that best meets your organization’s needs.
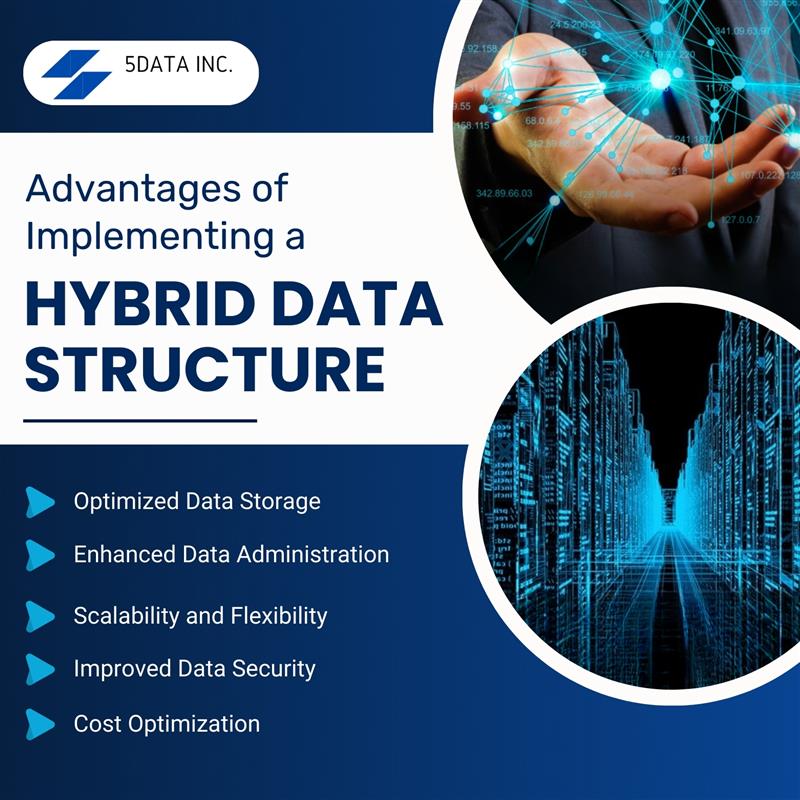
Advantages of Implementing a Hybrid Data Structure
- Optimized Data Storage: With a hybrid approach, organizations can store large volumes of data in data lakes while maintaining organized data in data warehouses for quick access and retrieval.
- Enhanced Data Administration: A hybrid model improves data quality, governance, and accessibility, ensuring that business users, data analysts, and data scientists can efficiently work with data.
- Scalability and Flexibility: Hybrid architectures support both traditional data retention and modern big data solutions, allowing organizations to scale resources based on data handling demands.
- Improved Data Security: Organizations can enforce strict data security policies across different data types and storage locations, ensuring the protection of sensitive data.
- Cost Optimization: Businesses can reduce costs by utilizing cloud storage for large-scale raw data while maintaining structured databases for high-performance computing.
Leading data collection and data management service providers offer seamless solutions for organizing, storing, and analyzing data to drive business success.
Use Cases for Hybrid Data Models
- Data Intelligence and Data Science Support both organized data reporting and data science on raw data.
- Machine Learning and AI: Provides data scientists with access to raw data required for model training and predictive analysis.
- Data Consolidation Across Cloud Platform: Ensures seamless data availability and synchronization across multi-cloud and on-premises infrastructure.
- Past Data Management: This technology enables businesses to store and analyze large volumes of past data for trend analysis and forecasting.
A reliable Application development service provider delivers custom software solutions tailored to meet business requirements and enhance user experiences.
Challenges and Opportunities
Implementing a hybrid data Model presents challenges such as data silos, integration complexities, and potential performance issues. To address these challenges, organizations should adopt a clear strategy that includes:
- Data Consolidation Complexities: Use automated ETL (Extract, Transform, Load) processes and data integration tools to streamline data ingestion and processing.
- Managing Data Quality: Implement robust data handling frameworks to maintain accuracy and consistency across organized and unorganized data.
- Ensuring Data Security: To safeguard sensitive information, adopt strict access control policies, implement encryption, and implement compliance protocols.
- Performance Optimization: Utilize caching, indexing, and query optimization techniques to enhance data extraction efficiency.
Best Practices for Implementing a Hybrid Data Models
- Define Clear Data Organization Policies: Ensure data integrity, compliance, and security across all data retention solutions.
- Leverage Cloud-Based Data Bank: Utilize data cloud systems for scalability and cost efficiency.
- Implement Data Cataloging: Maintain metadata and data lineage tracking to improve data accessibility and usability.
- Optimize Compute Resources: Allocate processing power based on data processing workloads to enhance performance.
- Enable Business User Accessibility: Provide intuitive tools and dashboards for non-technical business users to access and analyze data.
Efficient Data Life Cycle Management Services ensure secure data management, spanning from collection and storage to analysis and processing.
Future Trends in Hybrid Data Structures
- Increased Adoption of Data Lakehouses
- AI-Powered Data handling and Automation
- Enhanced Data Security Measures for Cloud Storage
- Expansion of Edge Computing for Real-Time Data Processing
Conclusion
Implementing a hybrid data model is essential for organizations that require a balance between organized data retention and flexible data analytics. By combining the best aspects of data lakes and data warehouses, businesses can enhance data management, optimize costs, and improve decision-making through predictive analytics and artificial intelligence capabilities. As data volumes continue to grow, hybrid data structures will be essential in defining the future of data-driven organizations.
Frequently Asked Questions
How does a hybrid data architecture improve business analytics?
A hybrid approach allows organizations to utilize structured and unstructured data for comprehensive business analytics, enabling better decision-making and analytics.
What are the key differences between a data lake and a data warehouse?
A data warehouse stores organized data with predefined schemas for fast queries, while a data lake stores raw, semi-structured, and unstructured data for flexible processing and analytics.
How does a hybrid data framework optimize costs?
It reduces storage expenses by keeping raw data in cost-effective data lakes while maintaining structured, frequently accessed data in high-performance databases.
What industries benefit the most from hybrid data structures?
Industries such as finance, healthcare, retail, and technology benefit from a hybrid data structure because they need both structured data intelligence and data science.
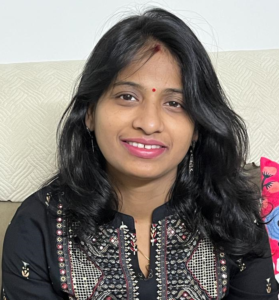
Rasmita Patro
Author