In order to thrive and excel in the dynamic domains of technology and business, staying informed about the latest advancements and trends is imperative. The terms “data science” and “data analytics” have become the buzzwords that often overlap and interconnect. Understanding the nuances and synergies between these two domains is crucial for professionals seeking to harness the power of data for making informed decisions.
This comprehensive guide aims to explore the intersection of data science and data analytics, shedding light on their link, the possibility of concurrently practicing both, transitioning from one to the other, and how professionals in these fields collaborate to extract insights. Professionals leverage tools like Power BI to analyze market trends, customer behavior, and resource allocation, replacing traditional methods with innovative approaches for insightful decision-making.
Link Between Data Science And Data Analytics
- Data Analytics: Data analytics is the exploration of extensive datasets to reveal concealed patterns and valuable insights. This method includes scrutinizing, cleaning, transforming, and modeling data to extract insights, propose conclusions, and facilitate decision-making. Through this process, businesses gain insights and extract a clear understanding of their data, enabling them to make more informed and strategic choices.
- Data Science: Data Science is a broad field that covers a range of disciplines, utilizing advanced algorithms, statistics, methods, and domain-specific knowledge to extract valuable perceptions from data. It encompasses the entire data lifecycle, including collection, cleaning, analysis, and interpretation. Data scientists leverage machine learning, artificial intelligence, and algebraic modeling to identify patterns, predict future trends, and derive actionable insights. The discipline plays a crucial role in informing strategic decision-making, uncovering hidden connections within massive datasets, and driving innovation across various industries.
The Overlapping
The intersection of data science and data analytics lies in their shared objective of extracting meaningful insights from data. Data science is the overarching umbrella that includes data analytics as a crucial component. Analytics is generally regarded as a subset of data science, focusing specifically on examining historical data to identify trends, patterns, and anomalies.
Balancing Data Science and Data Analytics
Individuals can effectively engage in both Data Science and Data Analytics, as these fields share fundamental skills while offering distinct perspectives on data. Data Analytics primarily involves interpreting historical data to uncover patterns and generate actionable insights. Professionals in this role often employ statistical analysis, exploratory data analysis, and data visualization.
On the other hand, Data Science is a broader field that includes analytics but extends to predictive modeling, machine learning, and advanced algorithm development. While Data Scientists delve into historical data, they also focus on creating Predictive analytics, utilizing artificial intelligence, and identifying future trends.
Overlapping Techniques
Professionals often find themselves using exploratory data analysis, statistics analysis, and predictive analytics, regardless of their primary role. Transitioning from Data Analytics to Data Science involves acquiring additional skills such as machine learning and a good command over scripting languages such as Python or R.
Continuous learning through courses or workshops can facilitate this transition, allowing individuals to harness the power of both fields. Ultimately, mastering both big data Science and Data Analytics provides a comprehensive skill set, enabling professionals to navigate the diverse challenges of the data landscape and contribute effectively to data-driven decision-making processes.
Yes, it is entirely feasible to engage in both big data science and data analytics, given the versatile skillsets required for each. Data analysts typically possess strong skills in statistical analysis, exploratory data analysis, and data visualization. On the other hand, data scientists often have a more extensive skill set, including machine learning, predictive modeling, and advanced algorithm development.
Data Collection and Data Management Service Providers play a pivotal role in streamlining the intricate process of handling large volumes of information. These specialized services ensure businesses can focus on leveraging Valuable perceptions while experts manage the complexities of collecting and organizing data.
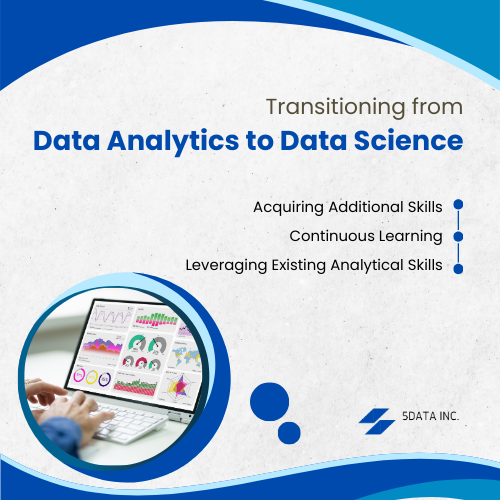
Transitioning from Data Analytics to Data Science
- Acquiring Additional Skills: To transition from Data Analytics to Data Science, professionals can follow a strategic planning approach focused on skill enhancement and continuous learning. Acquiring additional skills is paramount, encompassing proficiency in machine learning, a grasp of complex algorithm problems, and mastering programming languages like Python or R commonly used in data science.
- Continuous Learning: Engaging in continuous learning through online courses, workshops, or formal education programs serves as a bridge between data analytics and data science. Delving into predictive modeling, artificial intelligence, and mathematical modeling during this process expands the skill set and opens new opportunities within the broader field of data science.
- Leveraging Existing Analytical Skills: Importantly, leveraging existing analytical skills is a solid foundation for this transition. Data analysts can capitalize on their proficiency in identifying patterns and extracting insights from historical data, building upon these capabilities to incorporate more advanced techniques and methodologies associated with big data science. This strategic evolution allows professionals to seamlessly navigate the transition, combining analytical expertise with cutting-edge data science methodologies for a well-rounded skill set in the ever-evolving landscape of data-driven decision-making.
Data Analysts and Data Scientists Collaboration
- Complementary Roles: Data analysts and data scientists often work together collaboratively, each bringing their unique skills to the table. While data analysts focus on understanding and interpreting historical data to provide actionable insights, data scientists contribute by developing predictive models, employing machine learning algorithms, and identifying future trends.
- The Workflow: The workflow typically involves data analysts gathering and cleaning data, performing exploratory data analysis, and generating initial insights. Data scientists then take these insights to build predictive models, perform advanced analyses, and derive deeper insights that contribute to strategic decision-making.
- Bridging the Communication Gap: Effective collaboration requires clear communication between data analysts and data scientists. Bridging the gap between these two roles involves ensuring that the insights derived from data analytics are translated into actionable recommendations and that the technical aspects of data science are communicated in a way that is understandable to non-technical stakeholders.
Data Life Cycle Management Services offers comprehensive solutions for businesses to handle data efficiently from its creation to disposal. These services encompass data collection, storage, processing, and secure destruction, ensuring a seamless and compliant life cycle. By entrusting data life cycle management to specialized providers, businesses can optimize their data workflows and enhance overall operational efficiency.
Conclusion
The synergy of data science and analytics creates a dynamic realm, allowing professionals to seamlessly navigate roles, extract valuable insights, and make informed decisions. Whether one is primarily a data scientist, data analyst, or both, the evolving landscape of technology and business demands a holistic approach that embraces the versatility and overlapping techniques inherent in these domains. Continuous learning, collaboration, and a commitment to staying abreast of industry trends are essential for thriving in this exciting and ever-evolving field.
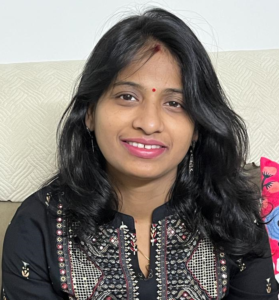
Rasmita Patro
Author