Key Takeaways
- Predictive analytics and AI drive smarter decisions by analyzing historical data to forecast trends and improve customer satisfaction.
- AI enables operational efficiency across industries, such as healthcare, finance, retail, and logistics, by optimizing resources and reducing risks.
- Digital transformation accelerates with AI-powered insights, promoting agility, data-driven cultures, and adaptive business models.
Predictive Analytics and AI
Predictive analysis leverages past data to forecast outcomes, while AI mimics human intelligence for complex decisions. Often, predictive analytics relies on AI models and machine learning algorithms to make predictions more accurate and actionable. AI plays a crucial role in analyzing vast datasets and spotting complex patterns that might escape human analysts, enhancing prediction reliability.
Data is central to predictive analytics and AI, powering pattern recognition, trend forecasting, and informed decisions. With more data, better quality, and faster processing, actionable insights and automation possibilities are rapidly expanding across industries.
By converting raw data into insights via AI, analysis, and visualization tools, companies make quality, data-driven decisions.
Experience seamless app functionality with our Top Mobile Performance Testing Services, designed to optimize speed, responsiveness, and reliability across devices.
Core Applications of PA & AI Across Key Industries
PA and AI have transformative applications across key industries, enabling smarter decision-making, enhanced customer experiences, and operational efficiencies. Here’s a breakdown of some core applications:
- Healthcare :
- Predictive insights from patient data for diagnosis, treatment planning, and preventative care.
- Predictive analysis aids in resource allocation, patient scheduling, and optimizing operational efficiency in healthcare facilities.
- Financial Services:
- Leveraging AI-driven models for fraud detection, personalized investment strategies, and credit risk management.
- Banks use predictive models to mitigate risks and enhance client satisfaction.
- Retail and E-Commerce:
- Using customer behavior data analysis to anticipate purchase trends, forecast demand, and optimize inventory levels.
- Predictive analytics enables personalized market trends and improves customer experience.
- Manufacturing:
- Optimizing production efficiency and quality control with predictive models.
- The role of AI is to identify patterns of chain bottlenecks, predict equipment failures, and reduce downtime.
- Logistics and Transportation:
- AI-driven route optimization, predictive maintenance, and demand forecasting to convert delivery times and reduce operational costs.
- Examples of how logistics companies use predictive analytics for better resource allocation and fuel efficiency.
- Telecommunications:
- Enhancing customer experience through proactive maintenance, trend prediction, and efficient resource deployment.
- Uses predictive models to anticipate network needs and customer behavior, improving service quality.
With our Functional Testing Services, we ensure your applications operate as intended, delivering reliable functionality across all features and scenarios.
Accelerating Digital Transformation Across Industries
Digital transformation is reshaping how industries operate, streamline processes, and deliver customer value by integrating digital technologies into every aspect of their business. Here are the core ways in which digital transformation is accelerating across various industries:
Customer Data and Behavior Insights
- Customer Segmentation: Data analytics uses behavioral data to segment customers for targeted marketing.
- Improving Customer Satisfaction: Uses predictive insights to anticipate customer needs and personalize offerings.
- Real-Time Customer Analytics: Companies use real-time data to adjust service offerings and ensure satisfaction.
Using Predictive Analytics for Better Operational Efficiency
- Inventory Management: Leverages AI for demand forecasting to optimize stock levels and reduce waste.
- Resource Allocation: Predictive models help companies allocate resources effectively based on past demand patterns and forecasted needs.
- Risk Management: Uses predictive analytics to anticipate risks and make proactive adjustments in operations and planning.
Actionable Insights and Forecasting Upcoming Events
- Market Trends Analysis: AI-driven predictive analytics enables businesses to monitor and respond to market shifts and trends.
- Anticipating Customer Preferences: AI models analyze past behavior to predict future preferences and buying habits.
- Predicting Upcoming Events: Predictive models help companies prepare for and mitigate potential disruptions.
Driving Digital Transformation with AI and Predictive Analytics
- Impact Across Industries: AI enables faster, more accurate, informed decisions to process across healthcare, finance, retail, and logistics.
- Enhanced Agility in Business Operations: AI and data analytics support agile responses to market changes, improving overall business adaptability.
- Supporting a Data-Driven Culture: Integrating AI into decision-making promotes a culture where data-driven insights are key strategic moves.
A leading Performance Testing Company ensures your applications run smoothly, handle high user loads, and deliver optimal speed and reliability.
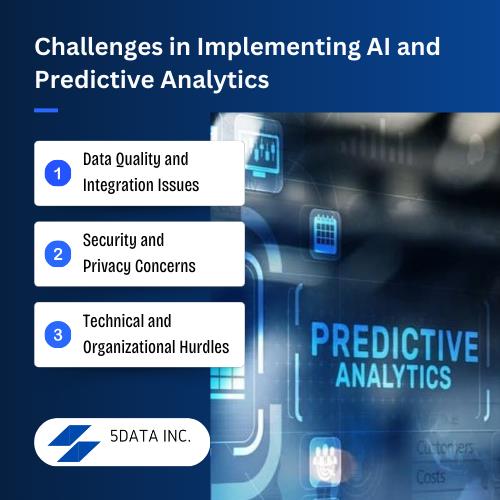
Challenges in Implementing AI and Predictive Analytics
Implementing AI and predictive analytics comes with notable challenges, which can be complex and resource-intensive. Here are a few challenges:
- Data Quality and Integration Issues: Difficulties in sourcing high-quality data and integrating it across systems.
- Security and Privacy Concerns: Data privacy issues are present in handling sensitive information, especially in sectors like financial institutions and healthcare.
- Technical and Organizational Hurdles: Need for skilled professionals, a supportive organizational culture, and technical infrastructure to implement AI systems effectively.
The Future of Predictive Analytics and AI
The future of predictive analytics and AI is driven by rapid advancements in ML, automation, and decision-making, promising growth, and enhanced outcomes across sectors.
- Ongoing Advancements: Enhanced machine learning models, increased automation, and AI’s role in more complex decision-making.
- Industry-Specific Innovations: Innovations in healthcare (like predictive diagnostics), retail (real-time demand forecasting), and finance (algorithmic trading).
- Towards More Inclusive AI: Companies are working on ensuring fairness and transparency in predictive models to avoid biases in data-driven decisions.
Conclusion
Predictive analytics and artificial intelligence provide organizations with a pathway to greater success by deepening their understanding of data and allowing for more informed, strategic decision-making. As these technologies continue to develop, businesses across different industries will increasingly adopt these solutions to anticipate outcomes, improve customer satisfaction, and maintain a market edge. The benefits of these innovations are significant, and the future looks promising, filled with opportunities for growth and improvement.
Frequently Asked Questions (FAQs)
1.What is predictive analytics, and how does it differ from AI?
Predictive analytics taps into statistical methods to review past data and forecast future outcomes, while AI takes things a step further, bringing together a range of technologies that allow machines to simulate human intelligence.
2.How does AI improve decision-making in business operations?
Artificial intelligence uses vast amounts of raw data to recognize patterns and trends, providing actionable insights that help companies improve processes, allocate resources, and enhance consumer satisfaction.
3.What are some common challenges companies face when implementing predictive analytics?
Challenges include ensuring data quality, addressing ethical considerations, integrating AI systems with existing infrastructure, and the necessity for skilled professionals to effectively manage data insights.
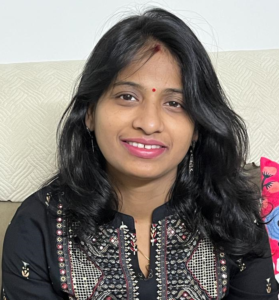
Rasmita Patro
Author