Data has become a valuable asset for organizations across industries in the modern digital age. Technological advancements have made collecting and storing vast amounts of raw data easier. However, transforming this raw data into meaningful and actionable insights is important for real-time analysis. Unlocking this value hinges on the utilization of data analytics. By applying various analytical techniques, organizations can uncover valuable information, identify patterns, trends, and correlations, and deeply understand operations and marketing efforts.There are several tools available to convert real-time data into actionable insights. Microsoft Excel offers basic data analysis features, while Tableau and Power BI provide powerful data visualization software. Python and R are popular programming languages with extensive data analytics and visualization libraries. Google Analytics is used for web analytics, Apache Hadoop enables distributed processing of large datasets, and SAS offers advanced actionable analytics and business intelligence functionalities.Unlock your business’s potential today with Data Life Cycle Management Services that leverage cutting-edge technologies, deliver valuable insights, and ensure seamless data operations. Choose the right tool based on analysis complexity, dataset size, data visualization needs, and specific project requirements.
What Is The Difference Between Data And Insights?
DataData refers to raw, unprocessed facts, figures, measurements, observations, or information. It represents the most fundamental level of information and can take various formats, such as numbers, text, images, or audio. Data, in isolation, lacks context and meaning. It is typically collected from different sources and can be structured or unstructured.InsightsInsights, on the other hand, are derived from data through analysis and interpretation. They represent meaningful, valuable, actionable insights that can change data-driven decisions. Insights result from extracting patterns, relationships, trends, or correlations from data, providing a deeper understanding of a specific phenomenon or problem.Key Differences
Context and meaning: Data lacks context and meaning in its raw form, context, and meaning. Insights provide a contextual understanding of the data by extracting patterns, trends, or relationships.Actionability: Data itself does not inherently drive action. On the other hand, insights are actionable information that can guide decision-makers and influence outcomes.Interpretation: Data requires interpretation and analysis to transform it into insights. Insights result from analyzing information and making sense of the data, providing a comprehensive understanding through data visualization or interactive dashboards.Level of abstraction: Data is concrete and represents specific information points. On the other hand, insights are more abstract and represent synthesized knowledge derived from big data.Purpose: Data serves as the foundation for generating insights. Insights are the desired outcome of data points, providing organizations with valuable knowledge to make informed choices and drive improvements.Data is the raw material, while insights are the refined and valuable output from business data. Insights provide a deeper understanding, identify patterns, and offer actionable guidance, allowing organizations to make knowledge-based decisions and drive positive outcomes. In the technology-driven world, data science is essential. Data Collection and Data Management Service Providers enhance data mining by implementing effective practices.How Do I Turn Data Into Actionable Insights?
The following steps can guide the interpretation and analysis of data into actionable insights:1. Define clear objectives
Clearly articulate the goals and questions the statistical analysis aims to address. Take, for example, key performance indicators. The metric cannot be deemed an insight if you don’t know how to respond to a certain KPI.2. Identify relevant data
Determine the data types most relevant to achieving the objectives.3. Clean and prepare the data
Ensure data accuracy and reliability by addressing missing values, inconsistencies, and errors.4. Apply data analysis techniques
Select appropriate techniques such as descriptive statistics, data visualization, or regression analysis to explore patterns and relationships in the data.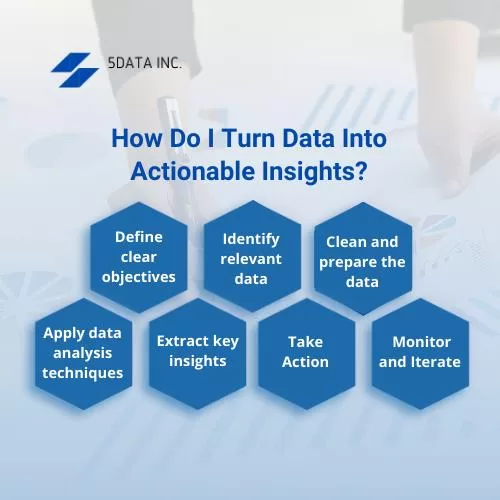
5. Extract key insights
The true power of analyzing data lies in its ability to extract actionable insights from the raw data. Actionable insights are specific, relevant, and practical information that can guide decision-making and drive positive outcomes. So, use some pie charts, bar charts, bubble charts etc. to make data insights accessible.6. Take action
Translate the actionable insight into specific actions, strategic decisions, or initiatives according to the marketing goals.7. Monitor and Iterate
Continuously assess the outcomes and refine the approach based on the results.Considering key factors like context, collaboration, long-term perspective, and ethical considerations throughout the process ensures that data-driven actionable insights empower informed decision-making and yield positive business outcomes.Can Today’s Software Find Actionable Insights In Data?
The answer is YES. Hire a Software Application Development Company to benefit from advanced analytics and artificial intelligence. They employ strong algorithms to analyze data, find patterns, and drawing conclusions, allowing you to stay ahead in marketing automation. They provide numerous strategies for locating important information such as-Data Exploration and Visualization: Interactive dashboard visualizations enable users to convert data intuitively, facilitating the identification of actionable insights through graphical representations.Statistical Analysis: Software tools provide various statistical techniques, such as regression analysis and clustering, to uncover meaningful relationships and significant findings within the data.Data Modeling: Data modeling involves creating mathematical or statistical models to predict future outcomes or analyze complex relationships within the data. ML algorithms are mostly used to build predictive models.Machine Learning and Predictive Analytics: Advanced software tools incorporate ML algorithms to learn from historical data and make predictions or recommendations based on patterns and trends. These predictive analytics capabilities help identify opportunities, forecast future outcomes, and support decision-making.Natural Language Processing (NLP): NLP techniques enable the analysis of unstructured data, extracting relevant insights from text sources and supporting strategies such as sentiment analysis or trend identification.Automated Data Mining: Software tools automatically explore large datasets, saving time and effort by turning raw data into insights and identifying hidden relationships and patterns.While software tools enhance the turning data process, human interpretation, domain expertise, and critical thinking remain essential to contextualize insights within the business context and make informed decisions.Conclusion
In today’s data-driven world, the power of data analysis cannot be understated. Organizations can unlock valuable insights, discover trends, optimize performance, and make informed decisions by analyzing raw data. Optimize your development process with a Top Application Development Company that prioritizes workflow efficiency through low-code automation and automated code testing. Because embracing data exploration as a core competency is essential for organizations aiming to remain competitive in digital transformation.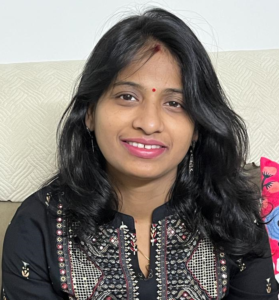
Rasmita Patro
Author