Key Takeaways
- Combining deep learning and data science improves data analysis and predictive modeling.
- These technologies excel in healthcare, customer analytics, and computer vision.
- Deep learning enhances the ability to extract meaningful insights from complex datasets.
- Data science and deep learning together improve the accuracy and depth of data mining.
- Emerging trends like Explainable AI and AutoML advance deep learning and data science.
Decoding Data Science And Deep Learning
Data science covers the complete data lifecycle, including collection, cleaning, analysis, and interpretation. Data scientists who drive this process utilize statistical techniques, programming languages, and specialized knowledge to derive valuable information from data. This information can be both structured and unstructured data, like customer records or financial data, or unstructured, like text, images, and audio.
Deep Learning is a division within machine learning models influenced by the organization and operations of the human brain. It utilizes artificial neural architectures, complex algorithms with multiple layers, to learn from data without explicit programming. These models excel at automatically identifying patterns and relationships within intricate datasets, demonstrating particular proficiency in processing unstructured training data.
The Synergy Between Data Science And Deep Learning
AI and data science provide the framework for data exploration, analysis, and interpretation, providing valuable insights. At the same time, deep learning models act as powerful tools for pattern recognition and uncovering hidden insights. This synergy offers several advantages:
- Extracting Meaning from Complex Data: Deep structured learning excels at interpreting unstructured data, such as social media posts, images, and videos. AI and Data Science integrate these insights with structured data for a comprehensive understanding. Data science techniques, combined with learning models, significantly improve the accuracy and depth of data mining. Data scientists use Deep structured learning to analyze complex datasets, whether structured or unstructured, extracting valuable insights that traditional methods struggle to achieve.
- Advanced Data Analytics: Machine learning algorithms can automate feature extraction and perform complex analyses that would be time-consuming or even impossible with traditional data science techniques. Deep Learning excels at uncovering underlying patterns within large datasets. By applying deep learning algorithms to data, data scientists can identify correlations and trends that are not immediately apparent.
- Predictive Modeling: Predictive analytics is a core aspect of AI and data science, involving the use of historical data to predict future outcomes. Machine learning improves predictive analytics by facilitating the creation of advanced and precise predictive models. These models can analyze data patterns effectively, proving indispensable in industries like finance, healthcare, and marketing, where precise forecasts are vital.
- Natural Language Processing (NLP): Machine learning has brought a revolution to natural language processing (NLP), allowing machines to understand human language with unprecedented accuracy. The integration of machine learning significantly enhances the capabilities of artificial intelligence in NLP.
Data Life Cycle Management Services ensure efficient handling of data from creation to deletion, optimizing storage, accessibility, and security throughout its lifespan.
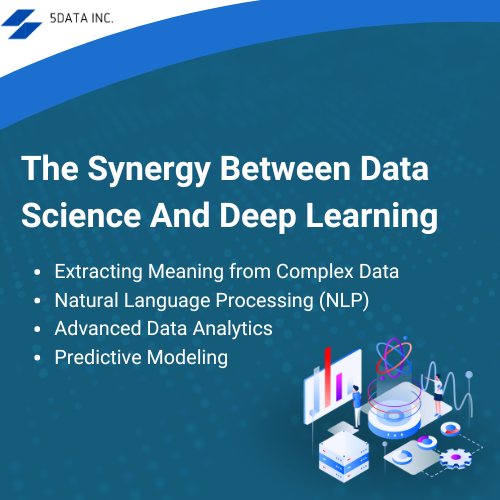
Data Science And Artificial Intelligence
The connection between artificial intelligence (AI) and Data Science is deeply interconnected. Artificial intelligence and data science provide the fuel (data) and tools (techniques) for AI systems to learn and evolve. Deep Learning models are a powerful example of how AI leverages data science principles to achieve intelligent behavior.
Data Science vs. Data Insights: While both data science and data insights deal with extracting knowledge from data, there’s a subtle difference. AI and Data science focus on the entire process, from data collection to model building. Data insights, on the other hand, emphasize the actionable information gleaned from data mining. Data scientists provide the tools and techniques, while data analytics translate the data-driven insights into actionable recommendations.
Advanced Analytics vs. Data Science: Advanced data analytics, focusing on intricate statistical models, machine learning algorithms, and data visualization tools, aims to unearth concealed patterns and predict forthcoming trends. In contrast, data science encompasses a wider array of tasks, including data cleaning, wrangling, and exploration, aimed at deriving actionable insights.
Applications Of Deep Learning And Data Science
As the synergy between machine learning and data science grows, so do the ethical considerations. Issues such as data privacy, algorithmic bias, the role data science techniques play, and the potential misuse of artificial intelligence systems must be addressed.
- Analyzing Customer Data: In the business world, examining data is crucial for understanding behavior, preferences, and trends. Deep learning models can process and analyze vast amounts of customer data, providing businesses with valuable insights to tailor their marketing strategies and improve customer satisfaction.
- Predictive Modeling in Healthcare: Predictive analytics can save lives in healthcare by anticipating disease outbreaks, predicting patient outcomes, and optimizing treatment plans. Deep Learning boosts these models by increasing accuracy and allowing machines to analyze complex medical data, leading to significantly more efficient resource allocation and markedly better outcomes in patient care.
- Enhancing Computer Vision: Computer vision, a field of artificial intelligence and data, relies heavily on machine learning to interpret and analyze visual data. Applications include facial recognition, object detection, and medical image analysis. By leveraging machine learning, data developers can develop advanced computer vision systems that perform tasks with high precision.
- Sentiment Analysis in Social Media: Social media generates vast amounts of unstructured data, which can be challenging to analyze. ML models enable data scientists to help businesses gauge public opinion, monitor brand reputation, and respond to customer feedback effectively.
Data scientists are poised to play a central role in upcoming developments. They will use their expertise to build and enhance models, interpret data, and offer actionable insights. Their proficiency in statistical modeling, handling complex datasets, and applying advanced data mining techniques will be essential in tackling the complexities and opportunities arising from the increasing volume of data. The Application Development Software Company excels in crafting bespoke solutions that address diverse client needs across various industries.
The Future Of Deep Learning And Data Science
As these fields mature, several exciting trends are emerging:
- Explainable AI (XAI): There’s a growing emphasis on developing ML models that are not just accurate but also explainable. Ensuring transparency is essential for fostering trust in AI systems and upholding their ethical deployment.
- AutoML (Automated Machine Learning): AutoML tools streamline the machine learning process by automating tasks like feature engineering, model selection, and hyperparameter tuning. They broaden access to AI and data science, making these fields accessible to users with limited technical expertise.
- Generative AI: Generative models learn to create entirely new data, such as realistic images, music, and even text. It has vast potential for applications in art creation, drug discovery, and material science.
The outlook for AI and data science is optimistic, driven by ongoing advancements in technology and methodology. As these fields evolve, they will unlock new possibilities for extracting meaningful insights from data. Innovations in DL, machine learning techniques, and data visualization will further enhance the capabilities of intelligence and data science, enabling more accurate predictions and better data-driven decisions. 5DataInc is a leading Mobile Application Development Company known for delivering high-quality, user-centric apps that cater to modern digital needs.
Conclusion
The convergence of deep structured learning and data science offers significant potential to improve our ability to derive valuable insights from intricate datasets. By harnessing the strengths of both disciplines, data scientists can uncover hidden patterns through thorough data analysis, enhance predictive modeling, and advance applications in artificial intelligence. As these technologies evolve and are further developed, the knowledge gained will foster innovation and enable informed decision-making across diverse industries, influencing future data-driven strategies.
Frequently Asked Questions (FAQS)
What are Data Science and Deep Structured Learning?
- Data science combines various techniques to extract meaningful insights from vast amounts of data, while Deep Structured Learning focuses on neural networks for complex tasks.
What is the difference between Data Science and Data Insights?
- Data science encompasses the entire data mining process, whereas data insights refer to specific findings or recommendations.
What is Deep Structured Learning in Data Analytics?
- Deep Structured Learning involves training neural net with multiple layers to handle complex data, such as images or text.
What is the difference between advanced Data Analysis and other Data Science models?
- Advanced data analysis extends beyond traditional and statistical analysis methods and includes machine learning algorithms and predictive modeling.
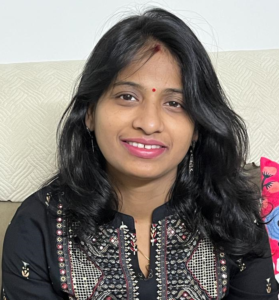
Rasmita Patro
Author